Cardiovascular Fitness
Pioneering New Methods for Fitness Assessment and Health Monitoring
​
We explore cutting-edge AI-driven methodologies for cardiovascular fitness assessment. By leveraging machine learning, wearable technology, and advanced signal processing, our work aims to revolutionize how we analyze and optimize human performance and well-being.
Our studies tackle the limitations of traditional fitness assessments, which often require expensive, invasive, and impractical lab-based testing. Through non-invasive, AI-powered techniques, we introduce novel approaches that enhance accuracy, accessibility, and personalization in health monitoring.​
​
Why This Matters
-
For Athletes & Trainers → Real-time, AI-driven insights for personalized training plans.
-
For Everyday Users → Wearable AI transforms fitness tracking into precision health monitoring.
-
For Healthcare Professionals → Non-invasive monitoring for patients with cardiac & metabolic conditions.​
Featured Research Areas
AI-Powered Cardiovascular Fitness Assessment
Cardiovascular fitness, often quantified by VOâ‚‚max, is a critical predictor of overall health and longevity. Traditionally measured through lab-based protocols, VOâ‚‚max can now be monitored dynamically using wearable sensors—ushering in a new era of personalized health tracking. But how can we go beyond static values and detect how our heart adapts to exertion in real time?
This page introduces two innovative studies that apply unsupervised machine learning—particularly k-means clustering and Dynamic Time Warping (DTW)—to heartbeat time series. These methods allow us to distinguish individual adaptation patterns during exercise and identify fatigue, stress, or efficient responses.
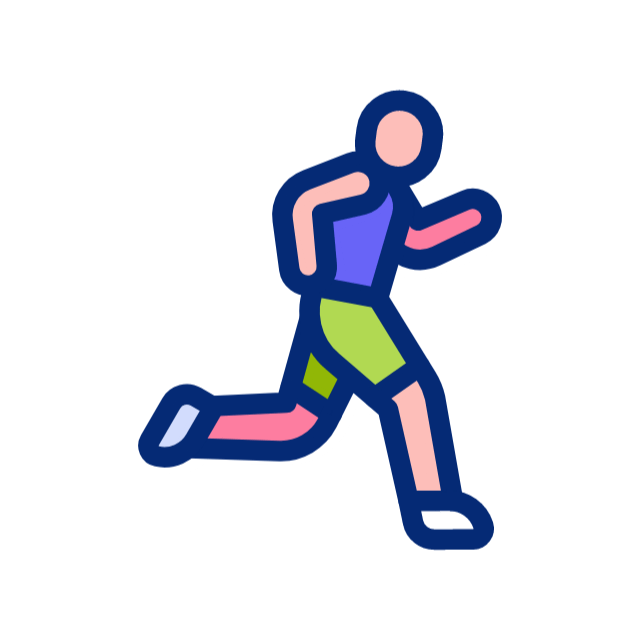
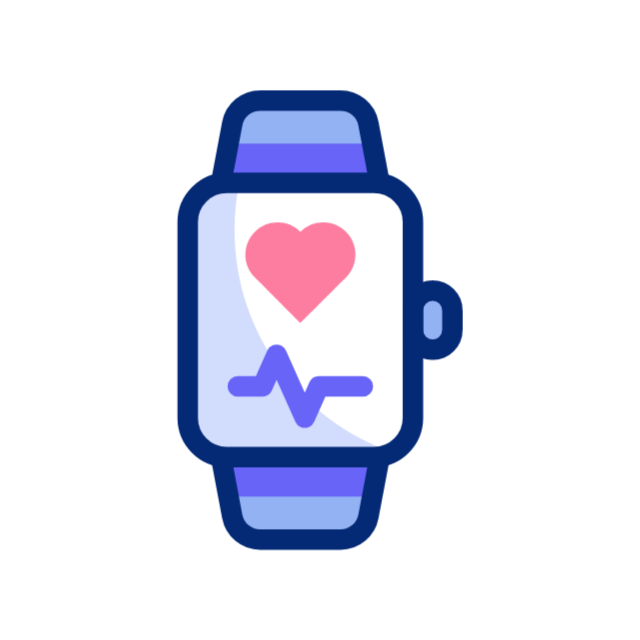
By clustering heartbeat, speed, and altitude variations in real-time, we identified four dynamic regimes during running, each associated with fitness improvements or signs of fatigue.
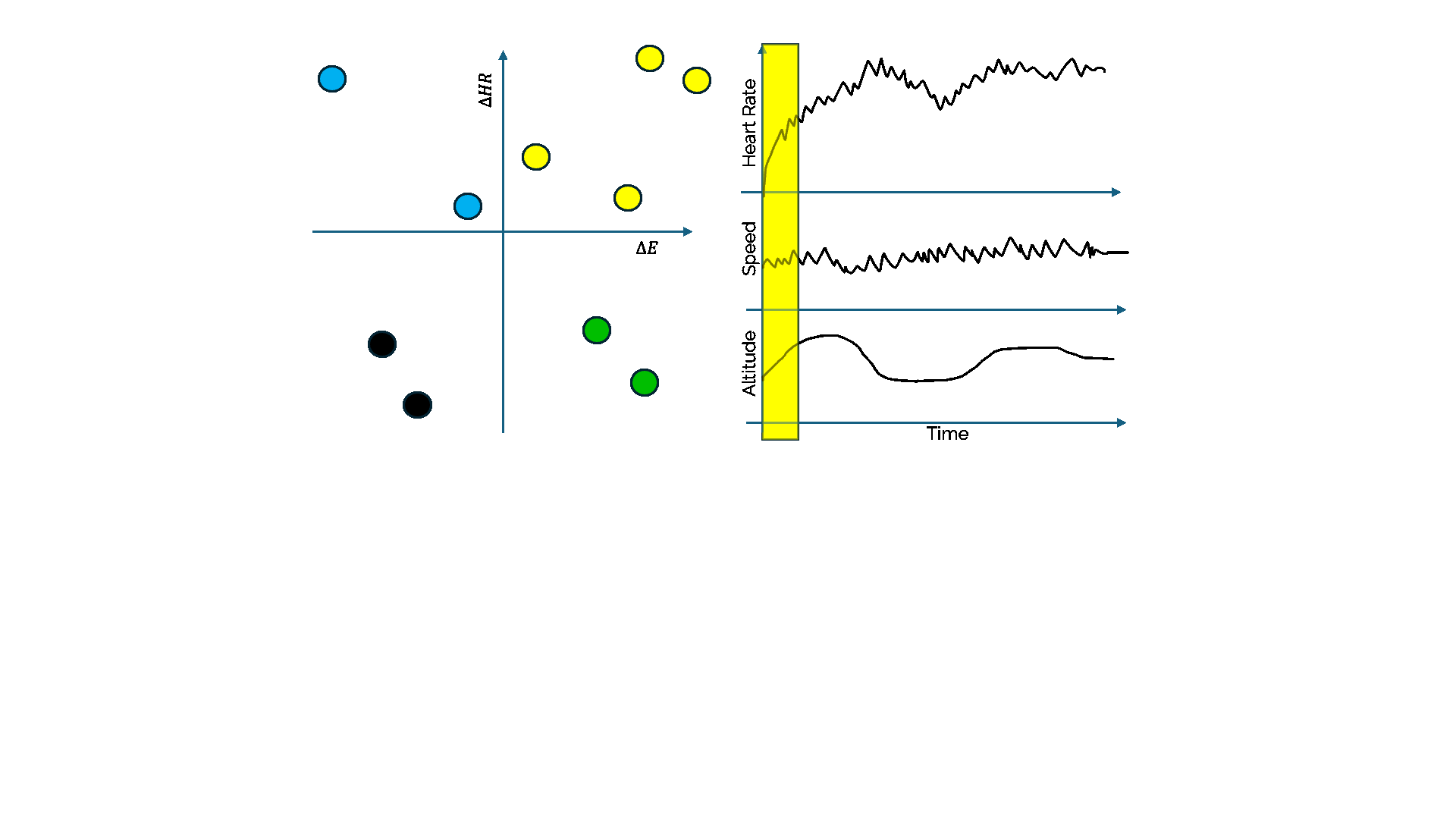
EA (Efficient Adaptation)🖤
AS (Active Strain) 💛
EI (Efficiency Improvement) 💙
BUP (Balance Under Pressure) 💚
Using data from 52 subjects performing a standardized step test, the study combines Dynamic Time Warping (DTW) and k-means clustering to classify trained vs. non-trained individuals and analyze how heart rate responses evolve across populations.-

These studies demonstrate how wearable tech and unsupervised AI can transform cardiovascular assessment. Instead of one-size-fits-all metrics, we can now offer dynamic, interpretable, and personalized feedback—turning raw heartbeats into actionable health insights.
Advancing Aerobic Threshold Detection with AI
At the forefront of exercise physiology and AI, our research aims to develop automatic, non-invasive methods for detecting the Aerobic Threshold (AerT) using Recurrence Quantification Analysis (RQA). This innovation eliminates the need for expensive and invasive procedures, making personalized fitness assessment more accessible than ever.
The Science Behind Our Work
What is the Aerobic Threshold?
The Aerobic Threshold (AerT) is the point where the body shifts from using aerobic metabolism (oxygen-based) to a mix of anaerobic metabolism, crucial for determining endurance levels and optimizing training programs.


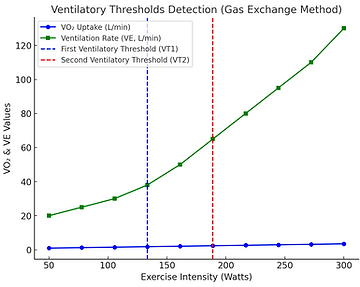

How Does RQA Improve AerT Detection?
​
Traditional methods require gas exchange analysis or blood lactate sampling, which are costly, complex and invasive. Indeed, blood lactate method determines thresholds by measuring lactate concentration in the blood during incremental exercise. This is done by taking a small blood sample is taken from a fingertip or earlobe every 3-5 minutes. Gas exchange analysis detects thresholds by analyzing oxygen consumption (VO₂), carbon dioxide production (VCO₂), and ventilation (VE) dynamics during exercise. The subject wears a metabolic mask connected to a gas analyzer during an incremental exercise test.​
Our approach:
​
- Uses heart rate time series recorded by wearable sensors.
- Applies Recurrence Quantification Analysis (RQA) to detect metabolic transitions.
- Provides real-time, automatic identification of AerT without manual interpretation.
Our latest research (2024) has confirmed that RQA is accurate across different age groups, health conditions, and training protocols, making it a versatile and scalable tool.
